Why Students Seek Help with their Data Mining Homework
Many data mining students often find themselves in a challenging predicament. Undoubtedly, navigating the maze of data mining homework can be a daunting task, requiring them to unearth patterns within vast datasets while constantly racing against the clock. If you find yourself buried under a mountain of data mining homework, our team of experts is ready to rescue you. We have a dedicated panel of in-house writers specializing in data mining homework help. Let's explore the key reasons why seeking assistance with data mining homework is essential for students:
- Time-Saving: Students typically have limited time to sift through extensive data sets and complete stacks of homework. Hence, data mining homework solutions become invaluable in conserving their time and energy.
- Improved Grades: Experts who provide data mining homework solutions play a pivotal role in helping students achieve high grades. When students receive guidance from these experts, they not only save time but also enhance their understanding of the subject, resulting in better performance in exams.
- Meeting Deadlines: Deadlines can be anxiety-inducing for students and may even negatively impact their grades. However, with experts taking full responsibility for completing their data mining homework, students can confidently submit their solutions on time.
- Plagiarism-Free Work: It's common for students to gather information from the internet when working on homework, which can inadvertently lead to unintentional plagiarism if not properly referenced. Experts, with their expertise, ensure that homework remain free from such issues.
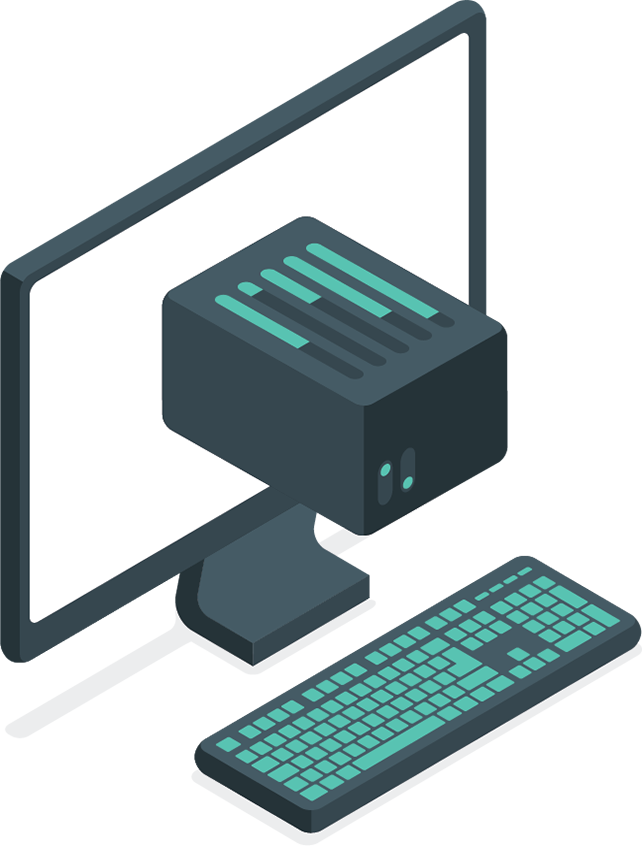
Get Your Data Mining Homework Done By Professionals At Affordable Rates
We understand the importance of affordable data mining homework help service. We believe that every student deserves access to quality help without financial strain. To achieve this, we have implemented a flexible pricing model that adapts to the individual needs and budgets of students. By customizing our rates based on the complexity, duration, and specific requirements of each homework, we ensure that our data mining homework help remains accessible and affordable. Our goal is to provide value-driven assistance that aligns with your financial capabilities, ensuring that cost never becomes a barrier to learning:
Service Type | Price Range |
---|---|
Basic Data Analysis | $20 - $50 (per homework) |
Advanced Data Mining Projects | $50 - $100 (per homework) |
Urgent Homework Assistance | $30 - $70 (additional urgency charge) |
Long-Term Project Support | $100 - $200 (per month) |
Data Visualization Help | $40 - $80 (per homework) |
Machine Learning Models | $60 - $120 (per homework) |
Big Data Analytics | $70 - $150 (per homework) |
Software-Specific Assistance | $30 - $70 (per homework, varies by software) |
We Offer Reliable Help with Data Mining Homework On All Topics
Our service caters to a diverse array of topics. We provide specialized help with all data mining homework. With a broad and deep range of expertise, we are uniquely positioned to assist students grappling with their data mining homework. Whether it's understanding complex algorithms or analyzing large datasets, our team of experts is equipped to offer tailored guidance and support. Our commitment is to ensure that every student receives the help they need to succeed in their data mining assignments, making even the most challenging concepts accessible and understandable.
Data Mining Homework Topics | Description of Expertise |
---|---|
Predictive Analysis | We specialize in teaching methods to analyze historical data for predicting future trends, using advanced statistical techniques and machine learning. |
Cluster Analysis | Our experts excel in segmenting data into distinct groups based on similarities, aiding in pattern recognition and decision-making processes. |
Association Rule Learning | We provide in-depth guidance on uncovering interesting relationships between variables in large databases, crucial for market basket analysis and more. |
Neural Networks and Deep Learning | Our team is adept at explaining complex neural network architectures and deep learning models, essential for advanced data mining tasks. |
Text Mining and Natural Language Processing | We offer expertise in extracting valuable insights from text data, utilizing NLP techniques to process and analyze large volumes of textual information. |
Anomaly Detection | Our service includes teaching methods to identify unusual patterns that deviate from the norm, critical in fraud detection and network security. |
Data Visualization and Interpretation | We provide skills in presenting data visually for easier understanding and interpretation, using various tools and techniques. |
Decision Trees and Random Forests | Our experts guide students in using decision tree algorithms and random forests for classification and regression, key in predictive modeling. |
Big Data Technologies | We cover the utilization of big data technologies like Hadoop and Spark for handling and processing large datasets efficiently. |
Time Series Analysis | Our team specializes in analyzing time-ordered data points to understand trends, seasonality, and cyclic behavior, crucial for financial and market analysis. |
We Have the Expertise to Complete Data Mining Homework Using Popular Tools
Data mining is the process of extracting valuable information from large datasets, transforming it into meaningful structures for further analysis and interpretation. It involves various techniques & tools from statistics, machine learning, and database systems to discover patterns and establish relationships in data, which can then be used to make informed decisions. Each of these tools brings unique capabilities to the table, and our expertise ensures that students can harness their full potential for their data mining projects.
- RapidMiner: This advanced analytics software is known for its ability to easily prep, model, and deploy data with rapid speed. We offer comprehensive guidance on how to use RapidMiner for complex data analysis and predictive modeling.
- Weka: A popular suite of machine learning software written in Java, Weka is ideal for data preprocessing, classification, regression, clustering, and visualization. Our experts can help you navigate its various features for effective data mining tasks.
- KNIME: Known for its user-friendly, graphical interface, KNIME allows for the creation of data-driven workflows. We provide assistance in utilizing KNIME for blending tools and data types, making data analysis more accessible and efficient.
- Python (with libraries like Pandas, NumPy, SciKit-Learn): Python is a versatile programming language with libraries that are crucial for data mining. Our service includes helping students leverage these libraries for data manipulation, statistical analysis, and machine learning applications.
- Tableau: As a leading data visualization tool, Tableau helps in transforming raw data into an easily understandable format. We offer expertise in using Tableau for creating insightful, interactive data visualizations that aid in making data-driven decisions.
- SAS (Statistical Analysis System): A powerful software for advanced analytics, SAS is widely used for data management, business intelligence, and predictive analytics. Our assistance covers how to effectively utilize SAS for complex data analysis and interpretation.
- Oracle Data Mining (ODM): A component of the Oracle Advanced Analytics Database, ODM offers powerful data mining capabilities. We help students understand how to use ODM for predictive analytics and data mining directly within the Oracle database.
- Microsoft SQL Server Analysis Services (SSAS): SSAS is a tool used for online analytical processing and data mining. Our experts guide students through its features for efficient analysis and interpretation of large datasets in a Microsoft SQL Server environment.
- Hadoop: An open-source framework, Hadoop is designed to store and process large datasets across clusters of computers. We provide insights on how to leverage Hadoop for distributed data storage and processing, crucial in handling big data.
- TensorFlow: TensorFlow is a free and open-source software library for machine learning and artificial intelligence. Our service includes teaching how to use TensorFlow for building and training neural networks, essential for advanced data mining tasks.
Sample Data Mining Homework & Projects Written By Our Experts
In our samples section, you will find a wide range of completed data mining homework and projects. These samples serve as an excellent reference for the quality of work we deliver. They cover various topics and complexities, showcasing the expertise and thoroughness of our team. By browsing through these samples, students can gain a clearer understanding of what to expect in terms of structure, depth of analysis, and presentation. These samples also offer insights into how different data mining concepts and tools are applied in real-world scenarios. Whether for inspiration or guidance, our samples are a valuable resource for students embarking on their data mining journey.