Data Mining Demystified: Simple Strategies for Successful Assignments
.webp)
In the rapidly evolving landscape of information technology, data mining has risen as a cornerstone discipline, playing a pivotal role in extracting invaluable insights from extensive datasets. As students venture into the intricate realm of data mining assignments, they are often met with the inherent complexities of the field, grappling with intricate concepts and methodologies. This dedicated blog aims to unravel the mysteries surrounding data mining, providing clear insights and presenting straightforward strategies to enhance success in data mining assignments. Acknowledging the challenges faced by students, the primary objective is to demystify the multifaceted nature of data mining, offering a comprehensive guide that empowers individuals to approach assignments with confidence. If you find yourself in need of assistance with your data mining homework, this blog is tailored to provide the support and insights required for success in your academic pursuits.
The intricacies of data mining often pose hurdles for students, creating a need for practical tools and knowledge to not only comprehend the nuances of the discipline but also excel in applying these concepts to real-world scenarios. This blog aspires to fulfill this need, breaking down the complexities into digestible components. Through this approach, readers are equipped with the resources necessary to navigate the often intricate landscape of data mining assignments. The overarching goal is to facilitate a smoother journey for students, fostering a deeper understanding and appreciation for the discipline's profound impact in the realm of information analysis and interpretation.
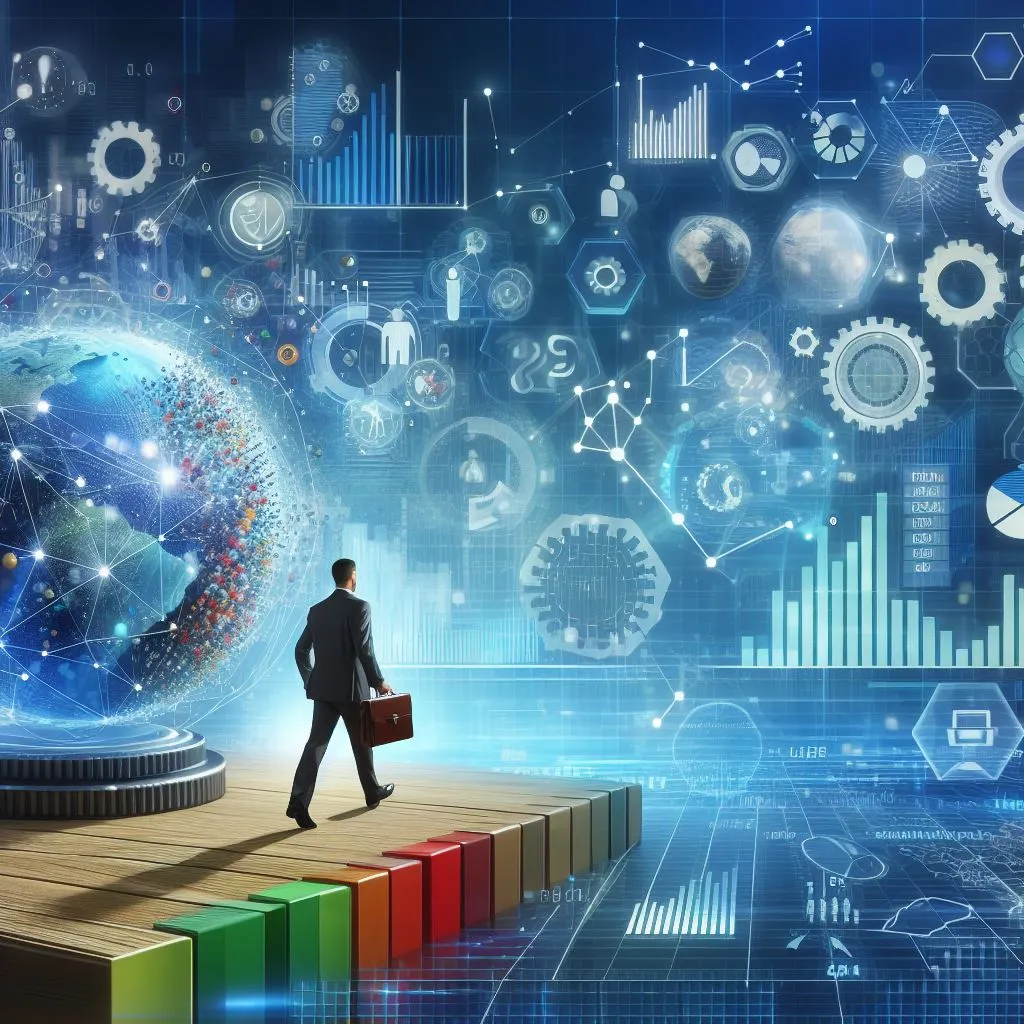
In essence, data mining is at the forefront of the technological revolution, guiding us through the vast sea of information and extracting meaningful patterns and insights. As we embark on this journey, it is crucial to recognize the transformative potential of data mining and its role in shaping the future of information technology.
Understanding the Basics of Data Mining
Embarking on the exploration of "Understanding the Basics of Data Mining," we delve into the intricate world of extracting valuable insights from vast datasets. In this comprehensive journey, we aim to demystify the foundational concepts that form the backbone of data mining. The term "Understanding" serves as our guiding principle, emphasizing a learner-centric approach as we navigate through the multifaceted landscape of this crucial discipline. Data mining, at its core, involves the art and science of uncovering patterns, trends, and relationships hidden within the vast expanses of data. It is a dynamic field that amalgamates techniques from statistics, machine learning, and database management, and this title sets the stage for unraveling the fundamental principles that underpin these methodologies.
As we delve into the basics, we lay the groundwork for comprehending the essence of data mining, breaking down complex concepts into digestible components. From understanding the significance of data warehouses as the bedrock for mining operations to grasping the role of algorithms as the engines driving pattern recognition and predictive modeling, this exploration aims to provide a holistic view of the essential elements within the data mining spectrum.
Moreover, we unravel key terminology integral to the data mining discourse. "Data Warehousing" takes center stage, and we navigate through the architecture and functionalities that enable efficient data retrieval and analysis. The discussion extends to algorithms, those intricate sets of instructions that fuel the data mining process. By comprehending their significance, we empower ourselves to navigate the diverse array of algorithms employed for various data mining tasks.
The overarching goal is not merely to introduce data mining concepts but to foster a deep and practical understanding. The content of this exploration is designed not as a mere theoretical exposition but as a bridge to practical applications. In the journey ahead, we will be peeling back the layers of data mining tools, exploring the intricacies of popular options like Weka, RapidMiner, and KNIME. By gaining hands-on experience, learners can seamlessly transition from theoretical understanding to practical implementation, bridging the gap between concept and application.
A significant facet of mastering data mining lies in the art of data preprocessing. This encompasses the cleaning and transformation of data to ensure its suitability for analysis. We navigate through techniques such as imputation, outlier detection, and normalization, understanding their importance in preparing data for effective mining. Feature engineering becomes our compass, guiding us to extract meaningful information from raw data, enhancing the predictive power of models.
Unraveling the Concept
Before delving into assignment strategies, it's crucial to establish a clear understanding of what data mining entails. At its core, data mining is the process of discovering patterns, trends, and relationships within large datasets. This intricate task involves employing a myriad of techniques from the realms of statistics, machine learning, and database management to systematically uncover hidden information that can prove invaluable in various domains.
Key Terminology Demystified
In the expansive world of data mining, certain key terms serve as linchpins to comprehension. One such term is "Data Warehousing," representing the foundation of this discipline. Data warehouses, organized repositories of data, play a pivotal role in facilitating efficient data retrieval and analysis. Another crucial facet is "Algorithms," the driving force behind data mining's pattern recognition and predictive modeling capabilities. Understanding these fundamental terms lays the groundwork for navigating the complexities of data mining with clarity and purpose.
Navigating the Data Mining Assignment Landscape
In the pivotal phase of tackling data mining assignments, a foundational element lies in effectively grasping the assignment objectives. To navigate this terrain successfully, students must first meticulously define clear objectives, a process that involves breaking down assignment requirements into specific, manageable goals. Understanding the nature of the task at hand is crucial – whether it pertains to classification, clustering, regression, or association rule mining. As the landscape of data mining assignments is diverse, the ability to identify key parameters emerges as the next critical step. This involves a nuanced exploration of essential parameters that are central to the assignment's goals. Such parameters may encompass the selection of appropriate attributes, the definition of target variables, and a profound comprehension of the significance inherent in the chosen dataset. Through these concerted efforts, students not only enhance their grasp of the assignment's core objectives but also lay the groundwork for a systematic and informed approach to the subsequent stages of data mining analysis. The mastery of these skills becomes an indispensable asset, empowering students to navigate the complexities of data mining assignments with clarity and purpose, ultimately contributing to the development of a robust foundation for analytical problem-solving in the broader context of data science..
Tools of the Trade: Choosing the Right Software
Delving into the expansive realm of data mining software presents an exciting journey of exploration, where understanding the landscape of popular tools becomes paramount for success. Navigating through widely embraced platforms such as Weka, RapidMiner, and KNIME offers a crucial starting point, each tool bringing its unique features, strengths, and limitations to the forefront. Weka, renowned for its versatility, empowers users with a vast array of algorithms for diverse data mining tasks, while RapidMiner stands out for its user-friendly interface and robust capabilities in predictive analytics. KNIME, with its open-source nature, provides a flexible environment for data manipulation and analysis. Amidst these options, gaining hands-on experience emerges as a linchpin for proficiency. The opportunity to delve into practical applications, guided by tutorials and sample datasets offered by these tools, becomes a cornerstone for users to traverse the learning curve and become intimately familiar with the intricacies of each platform. This hands-on experience not only solidifies theoretical knowledge but also cultivates a deeper understanding of the practical implications and nuances of data mining software. As users navigate this landscape, the synthesis of theoretical understanding and practical proficiency paves the way for a comprehensive mastery of data mining tools, enabling effective utilization in real-world scenarios and optimizing the potential for success in assignments and beyond.
Data Preprocessing: The Foundation for Success
Navigating the critical phase of data preprocessing, where the foundation for successful data mining assignments is laid, involves a meticulous exploration of two key components: Data Cleaning Techniques and Feature Engineering. In the realm of Data Cleaning Techniques, the focus is on addressing the pervasive issues of missing values, outliers, and inconsistencies that often plague datasets. This multifaceted process demands a strategic approach, delving into methodologies such as imputation to fill in missing data points, outlier detection to identify and manage data anomalies, and data normalization to ensure uniformity in scale and facilitate accurate comparisons. This segment serves as the bedrock for subsequent analyses, as the quality of insights drawn is inherently linked to the cleanliness of the dataset. Complementing this is the art of Feature Engineering, a practice that transcends the conventional boundaries of raw data. Here, the emphasis is on elevating the predictive power of models by either creating entirely new features or transforming existing ones. The essence lies in extracting meaningful information from the raw data, sculpting variables that not only enhance the model's performance but also contribute significantly to the overall understanding of patterns and relationships within the dataset. In this intricate dance between cleaning and crafting data features, practitioners unlock the potential for more accurate, reliable, and insightful data mining outcomes, ultimately shaping the success of assignments in this dynamic landscape.
Selecting the Right Model: A Critical Decision
Navigating the intricate realm of data mining enters a critical phase known as model selection, a juncture where decisions wield profound implications for the outcomes of analytical endeavors. In this pivotal stage, the onus lies on practitioners to sift through a myriad of strategies, each holding the potential to shape the trajectory of data-driven insights. The title, "Selecting the Right Model: A Critical Decision," encapsulates the essence of this journey, where the stakes are high, and precision is paramount. As we embark on this exploration, we find ourselves immersed in a landscape where the art and science of data mining converge. Model selection is not merely a procedural step; it is the fulcrum upon which the efficacy of analytical models pivots. The imperative nature of this decision-making process demands a nuanced understanding of diverse model types, each endowed with its own strengths, weaknesses, and applicability. Through the lens of this critical decision, we unravel the intricacies of decision trees, neural networks, support vector machines, and an array of other model archetypes that populate the data mining toolkit. The title serves as a beckoning guide into the heart of analytical decision-making, where the pathway to success requires not just technical proficiency but also a strategic grasp of the problem at hand. We traverse the terrain of model selection, illuminating the significance of aligning model types with the specific requirements of the data mining task, be it classification, clustering, regression, or association rule mining.
The journey unfolds further as we delve into the fabric of cross-validation techniques, a crucial companion in the model selection odyssey. Cross-validation serves as a litmus test for the robustness and generalizability of chosen models, ensuring that the selected model is not just adept at fitting the training data but is poised to excel in the face of unseen data. This juncture in the data mining narrative is marked by a fusion of theory and practice, where theoretical understanding finds resonance in practical application. The title, "Selecting the Right Model," is not a mere proclamation; it is an invitation to engage in the intricate dance between conceptual knowledge and hands-on experience. As we scrutinize the outputs of selected models, we navigate through matrices of accuracy, precision, recall, and F1 score, translating abstract metrics into tangible insights that guide the decision-making process. Visualization techniques emerge as allies in this endeavor, offering a visual narrative that complements the numerical precision of metrics, enhancing the communicative power of analytical findings.
In the expansive landscape of data mining, the title acts as a compass, guiding practitioners through the labyrinth of choices, trade-offs, and considerations inherent in the model selection phase. The critical decision at the heart of this exploration is not just about picking a model; it's about sculpting a solution that aligns with the nuances of the data and the intricacies of the problem domain. The title encapsulates the gravity of this decision, signaling to the reader that what follows is not just a technical discourse but a strategic dialogue about shaping the trajectory of analytical endeavors. The title, "Selecting the Right Model: A Critical Decision," is an ode to the pivotal moment where the path to successful data mining takes shape—a moment where expertise converges with intuition, and the right model becomes a beacon in the dynamic landscape of information discovery.
Understanding Model Types
A fundamental consideration lies in comprehending the diverse types of models tailored for specific data mining tasks. From decision trees, neural networks, to support vector machines, each model encapsulates unique strengths and weaknesses, thereby demanding a nuanced understanding to discern their appropriateness for distinct scenarios. This nuanced understanding is pivotal in aligning the chosen model with the specific objectives of the data mining assignment, ensuring that the analytical approach is not only effective but also tailored to the intricacies of the dataset at hand.
Cross-Validation Techniques
Another indispensable aspect of model selection involves the implementation of cross-validation techniques, a methodology that transcends the conventional approach of model evaluation. By integrating cross-validation, the assessment of the model's performance becomes a more comprehensive and rigorous process, enhancing its robustness and reliability. This technique involves partitioning the dataset into multiple subsets, iteratively training the model on different subsets and validating it on the remaining data. The iterative nature of cross-validation provides a more accurate reflection of the model's generalizability and minimizes the risk of overfitting or underfitting. As a result, practitioners gain a more holistic understanding of the model's predictive capabilities, allowing for informed decisions in the selection of the most suitable model for the given data mining task.
Delving into Strengths and Weaknesses
Careful consideration of model types and the incorporation of cross-validation techniques underscore the meticulous approach required in model selection, illustrating its pivotal role in the overarching success of data mining assignments. By delving into the strengths and weaknesses of each model type and embracing cross-validation as a robust evaluation tool, practitioners can navigate the intricate landscape of model selection with confidence, ensuring that their chosen model aligns seamlessly with the complexities of the dataset and the objectives at hand. In essence, model selection emerges not merely as a technical step in the data mining process but as a strategic decision that profoundly influences the quality and reliability of the insights extracted from large datasets.
Interpretation and Evaluation of Results
Navigating the intricacies of data mining outputs requires a holistic approach that extends beyond mere numerical analysis. Interpreting model outputs demands a comprehensive understanding of the contextual relevance of metrics like accuracy, precision, recall, and F1 score, enabling individuals to discern the practical implications of their findings. This nuanced interpretation forms the bedrock of informed decision-making, guiding users towards robust conclusions and actionable insights. Visualization techniques play a complementary role by providing a visual narrative of the data patterns uncovered. Whether through heatmaps, which vividly portray data density and distribution, scatter plots elucidating relationships between variables, or confusion matrices offering a clear snapshot of classification performance, the art of visualization amplifies the communicative power of data. Selecting the right visualization method is an art in itself, demanding an intuitive grasp of the dataset's intricacies and the underlying story it tells. As such, mastering the interplay between interpretation and visualization becomes a formidable skill, empowering individuals to not only understand the output of their data mining models but also to convey these insights in a compelling and accessible manner. This synergy ultimately transforms data from a collection of numbers into a narrative that informs decision-makers and stakeholders.
Staying Updated: The Dynamic Nature of Data Mining
In the ever-evolving landscape of data mining, the imperative of continuous learning stands as a beacon for those seeking mastery in this dynamic field. The vitality of staying informed on industry trends cannot be overstated. Data mining, as a discipline, experiences constant advancements propelled by technological breakthroughs and evolving methodologies. To navigate this intricate landscape successfully, individuals must conscientiously follow industry publications, attend conferences, and actively engage in online forums. These platforms serve as virtual hubs where the pulse of data mining innovation beats strongest, providing insights into the latest trends, emerging techniques, and best practices. The symbiotic relationship between theory and practice is fortified by the second prong of continuous learning: participation in online communities. Platforms such as Stack Overflow, Kaggle, and LinkedIn facilitate a collaborative environment where data mining enthusiasts converge to discuss challenges, seek advice, and share invaluable experiences. Through these interactions, individuals not only expand their knowledge base but also gain practical insights into real-world applications of data mining. Embracing continuous learning in this manner is not merely a recommendation; it is a fundamental strategy for staying relevant and thriving in a field that thrives on innovation and collective intelligence. As the data mining community evolves, those who actively embrace continuous learning will find themselves at the forefront of industry advancements, equipped with the skills and insights needed to navigate the ever-shifting landscape with agility and expertise.
Conclusion
In our exploration of the intricate realm of data mining, the path to success in assignments is unveiled as a multifaceted journey, reliant on a synthesis of core conceptual understanding, strategic tool selection, and the adept application of effective methodologies. Central to this expedition is the mastery of fundamental aspects, a dynamic fusion that empowers individuals to traverse the expansive landscape of data mining with unwavering confidence. Recognizing that success is woven into the fabric of comprehending core concepts, students are encouraged to unravel the complexities inherent in data mining assignments, transforming challenges into opportunities for growth and learning. The judicious selection of tools emerges as a pivotal factor, with an emphasis on not just familiarity but true mastery, ensuring that the chosen instruments align seamlessly with the task at hand. The application of effective strategies throughout the data mining process becomes a guiding beacon, illuminating the pathway to success and unveiling the hidden treasures within vast datasets. As this comprehensive approach takes root, individuals find themselves not only achieving success in assignments but also unlocking valuable insights, positioning them as adept navigators in the world of data mining. It becomes evident that the journey towards proficiency in data mining is an enriching odyssey in itself, transcending the mere destination of assignment completion. This realization prompts an appreciation for the profound impact of data mining on information analysis and interpretation. As individuals venture through this expedition, the journey becomes a celebration of learning, growth, and the empowerment that arises from mastering the intricacies of data mining. Thus, with a resounding acknowledgment of the transformative power embedded in understanding core concepts, selecting the right tools, and implementing effective strategies, the message resonates – the key to success in data mining assignments lies not merely in reaching the destination but in relishing the entire voyage. Happy mining!