The Role of AI in Modern Database Management
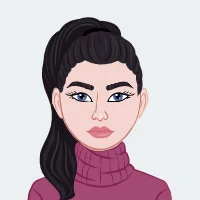
In the dynamic realm of technology, the integration of Artificial Intelligence (AI) into database management has emerged as a transformative force, fundamentally altering the way we handle, process, and derive insights from data. As technology advances at an unprecedented pace, the fusion of AI and database management transcends being a mere trend; it signifies a paradigm shift. This blog aims to unravel the profound impact of AI on modern database systems, delving into the intricacies, benefits, and challenges associated with this dynamic partnership. If you need assistance with your database homework, exploring the intersection of AI and database management provides a rich field for understanding the evolving landscape of data management and analysis, offering insights into how AI technologies can enhance database functionality and performance.
AI's influence on modern databases extends beyond mere automation; it revolutionizes the very core of data management. By harnessing advanced algorithms and machine learning, AI introduces a level of intelligence that transforms databases into adaptive, efficient, and insightful entities. One key area where AI excels is in automating routine database tasks, seamlessly handling activities like backups, updates, and maintenance. This automation not only ensures accuracy and reliability but also frees up valuable time for database administrators to focus on more strategic aspects of data management.
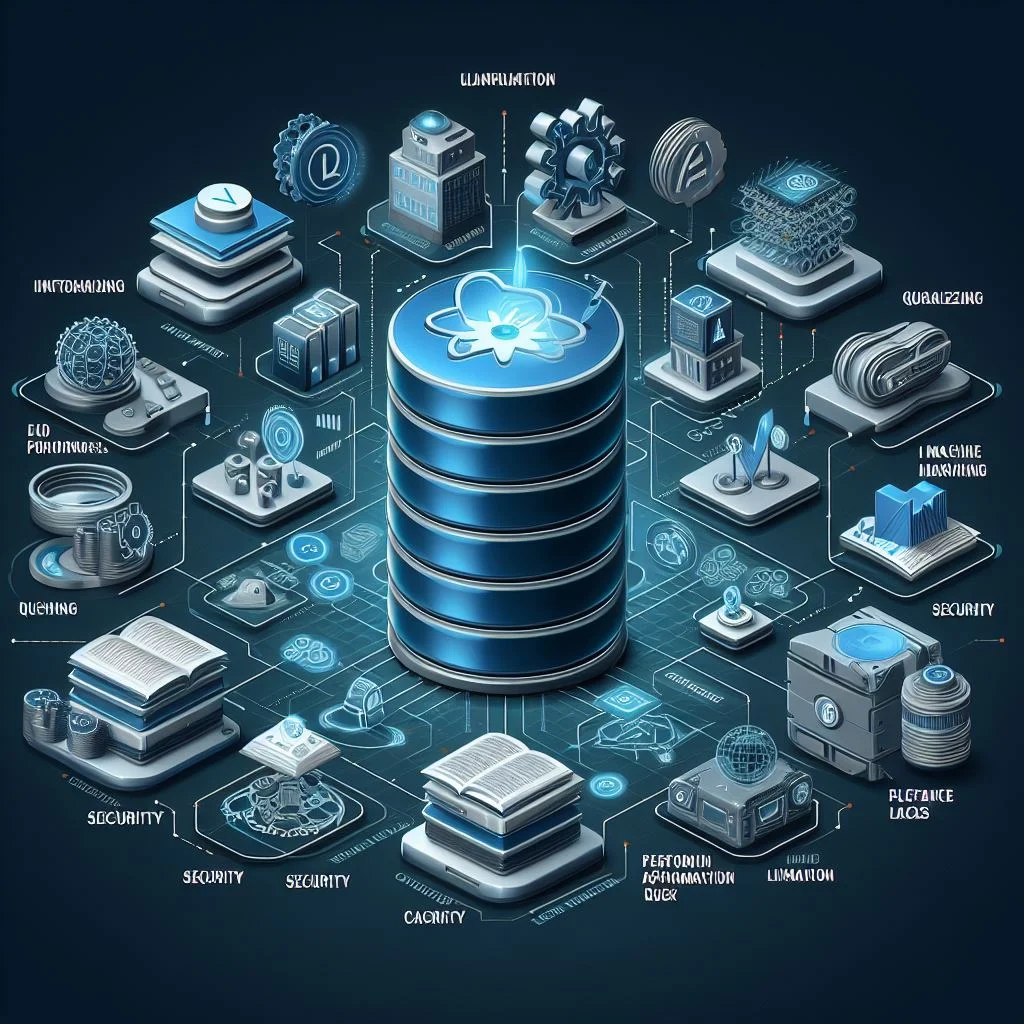
Furthermore, AI-driven query optimization stands out as a remarkable facet. Through the analysis of historical data usage patterns, AI algorithms enhance SQL queries, leading to faster and more efficient data retrieval. This not only improves overall system performance but also contributes to a more streamlined and responsive user experience. The transformative power of AI in databases lies not only in its ability to streamline operations but also in its capacity to uncover valuable insights from vast datasets.
However, integrating AI into database management is not without its challenges. Addressing concerns related to data privacy and managing the complexity of AI models are critical considerations. Encryption, robust access controls, and privacy-enhancing techniques play a pivotal role in mitigating privacy concerns. Simultaneously, efforts to simplify the deployment and maintenance of AI models are underway to make the integration more accessible to a broader audience.
The real-world applications of AI in database management span various industries and have a significant impact. In the healthcare sector, AI facilitates personalized patient care and accelerates medical research by extracting valuable insights from vast datasets. In e-commerce, AI optimizes databases to provide tailored product recommendations and refines customer relationship management strategies, enhancing the overall shopping experience. These applications showcase the versatility and practicality of integrating AI into diverse fields, promising increased efficiency and innovation.
Looking ahead, the synergy between AI and quantum computing holds immense promise. The advent of quantum computing could exponentially amplify AI's capabilities, ushering in a new era of data processing power. Additionally, the concept of autonomous databases, where AI takes the lead in managing, optimizing, and securing databases, is on the horizon. This promises a future marked by reduced human intervention and increased operational efficiency, paving the way for a new era in database management.
In conclusion, the integration of AI into modern database management is not just a technological trend; it's a paradigm shift. This dynamic alliance brings unprecedented efficiency, security, and innovation to the forefront, reshaping the landscape of data management as we know it. Join us on this exploration of AI's transformative role, where the intricate dance between algorithms and databases paves the way for a data-driven future.
Understanding the Basics: AI and Database Management
In the realm of database management, Artificial Intelligence (AI) is a revolutionary concept that brings a paradigm shift in how data is handled. Specifically, AI in the context of database management refers to the strategic application of advanced algorithms and machine learning techniques. The primary goal is to automate, optimize, and enhance various aspects of data handling, ultimately transforming the traditional database landscape.
As we embark on understanding the evolution of database management systems, it becomes imperative to trace their historical journey from conventional to modern paradigms. The traditional database management systems were characterized by their structured and static nature, often reliant on rigid schemas. However, with the exponential growth of data and the emergence of diverse data types, these traditional systems started facing limitations in terms of scalability, flexibility, and performance.
The need for AI integration into database management becomes evident as we confront the challenges posed by the escalating complexities of modern data. Unlike the structured data of the past, contemporary databases contend with an ever-expanding volume of unstructured and semi-structured data. The historical journey of database management systems reflects the necessity to evolve in response to these changing data dynamics.
In the early stages of database evolution, hierarchical and network models dominated the landscape. These models, while effective for certain applications, lacked the flexibility required to handle the evolving nature of data. The advent of the relational database management system (RDBMS) marked a significant shift, introducing a more flexible and scalable approach with its tabular structure and SQL querying language.
However, as data continued to grow in volume and complexity, new challenges emerged. NoSQL databases emerged as a response to these challenges, offering more flexibility to handle diverse data types and scalable architectures suitable for modern, large-scale applications.
Yet, even with the capabilities of NoSQL databases, the demands of real-time analytics, artificial intelligence, and machine learning applications pushed the boundaries further. This is where the integration of AI into database management becomes not just beneficial but imperative.
The evolving landscape of database management systems underscores the need for systems that can adapt to the dynamic nature of modern data. AI, with its ability to analyze patterns, automate routine tasks, and optimize queries, addresses these challenges effectively. Machine learning, a subset of AI, plays a pivotal role in predictive analytics, enabling databases to anticipate trends and optimize performance.
In essence, the historical journey from traditional to modern database management systems has been a quest for adaptability and efficiency in handling increasingly complex and voluminous data. AI integration is the next logical step in this evolution, providing the intelligence and automation needed to unlock the full potential of modern databases. As we continue exploring the intersection of AI and database management, we will uncover the diverse ways in which this integration revolutionizes data handling and shapes the future of information management..
AI-Powered Database Automation
In the intricate realm of database management, the integration of Artificial Intelligence (AI) has proven to be a powerful ally, particularly in streamlining routine tasks that have long consumed valuable time and resources. This section will explore how AI brings unprecedented efficiency to the table by automating mundane yet essential database tasks such as backups, updates, and maintenance. The overarching aim is to liberate database administrators from the burdensome aspects of day-to-day operations, allowing them to redirect their focus towards strategic decision-making.
Routine database tasks, despite their importance, often become time-consuming and repetitive for administrators. AI steps in as a transformative force by automating these tasks, bringing efficiency and precision to the forefront. For instance, the automation of backup processes ensures that data is consistently secured without manual intervention. Updates to databases, a critical aspect of keeping systems current and secure, are seamlessly executed by AI algorithms, reducing the risk of human error and downtime.
Maintenance tasks, which traditionally demanded meticulous attention to detail, can now be intelligently automated through AI. Whether it's optimizing data structures, purging redundant information, or ensuring system health, AI-driven automation allows for a proactive and streamlined approach. By alleviating administrators from the burden of routine tasks, AI empowers them to engage in more impactful endeavors—strategic decision-making that shapes the overall direction and efficiency of the organization's data management.
Delving into the intricacies of AI-driven query optimization unveils a world where algorithms become adept at not just processing but enhancing SQL queries for optimal performance. As databases grow in complexity and size, the efficiency of data retrieval becomes a critical factor in maintaining system responsiveness.
AI-driven query optimization operates on a proactive and adaptive model. Instead of relying solely on fixed query structures, algorithms analyze historical data usage patterns, adapting and enhancing queries based on the evolving needs of the system. This results in a significant boost to the speed and efficiency of data retrieval, a crucial factor in today's data-centric landscape where timely access to information is paramount.
The transformative impact of AI in query optimization goes beyond mere speed improvements. By intelligently enhancing queries, AI contributes to a more responsive and user-friendly database experience. This not only benefits internal stakeholders but also enhances the overall efficiency of applications and services relying on these databases.
In essence, the integration of AI in database management redefines the role of administrators, liberating them from routine tasks and enabling them to become strategic decision-makers. Simultaneously, AI-driven query optimization ensures that databases operate at peak performance, catering to the dynamic needs of modern applications. As we navigate through the various facets of AI in database management, it becomes evident that this symbiotic relationship holds the key to a future where data operations are not just automated but optimized to meet the demands of a rapidly evolving digital landscape.
Machine Learning in Database Management
In the ever-evolving landscape of database management, the integration of machine learning algorithms introduces a new dimension—predictive analytics. This section delves into how these advanced algorithms can predict future trends and performance patterns, revolutionizing database efficiency by allowing proactive measures to be taken to prevent potential issues.
Machine learning algorithms, when applied to databases, transcend the traditional role of data processing. They become powerful tools for foreseeing future trends and performance patterns, fundamentally altering the approach to database management. By analyzing historical data and recognizing patterns, these algorithms can make informed predictions about future data usage, system loads, and potential bottlenecks.
The proactive nature of predictive analytics allows database administrators to stay ahead of the curve. Instead of reacting to issues as they arise, administrators can anticipate and address potential challenges before they impact performance. For instance, if a surge in user activity is predicted, resources can be allocated or scaled up in advance to ensure seamless system operation. This predictive approach not only enhances overall database efficiency but also contributes to a more reliable and responsive user experience.
The realm of database security is one where the adaptive capabilities of machine learning truly shine. Traditional security measures often rely on predefined rules and signatures, struggling to keep pace with the dynamic landscape of emerging threats. Here, machine learning algorithms, as part of artificial intelligence, play a pivotal role in adapting and evolving to counteract these threats effectively.
Machine learning algorithms can continuously analyze patterns of user behavior and system activities, discerning normal from anomalous activities. This adaptability allows the algorithms to detect and respond to emerging threats in real-time, providing a robust layer of protection for sensitive data. Whether it's identifying unusual login patterns, detecting unauthorized access attempts, or recognizing patterns indicative of data breaches, machine learning enhances the proactive security posture of databases.
The adaptive security measures introduced by machine learning extend beyond traditional rule-based systems, offering a dynamic and evolving defense mechanism. As cyber threats become increasingly sophisticated, the ability of machine learning algorithms to learn and adapt ensures that databases remain resilient in the face of ever-changing security challenges.
In essence, the integration of machine learning into database management ushers in a new era of anticipatory and adaptive strategies. Predictive analytics enables administrators to forecast future demands on the database, allowing for proactive adjustments to optimize performance. Simultaneously, adaptive security measures, powered by machine learning, provide a dynamic defense against evolving threats, ensuring that sensitive data remains secure in an ever-changing digital landscape. As we navigate through these applications of machine learning in database management, it becomes clear that this technology not only enhances current operations but also fortifies databases for the challenges of tomorrow.
Challenges and Solutions in AI-Integrated Database Management
As the integration of Artificial Intelligence (AI) into database management progresses, addressing data privacy concerns becomes a paramount consideration. This section explores the challenges associated with AI handling sensitive data and discusses key strategies, including encryption, access controls, and privacy-enhancing techniques
One of the chief concerns in leveraging AI for database management revolves around the handling of sensitive data. As AI algorithms delve into vast datasets to extract valuable insights, ensuring the privacy and security of this information becomes critical. Encryption emerges as a fundamental safeguard in this context, where sensitive data is transformed into an unreadable format, accessible only to authorized entities.
In addition to encryption, robust access controls play a pivotal role in mitigating data privacy risks. Implementing stringent access policies ensures that only authorized personnel can interact with and extract information from the database. Fine-grained controls based on user roles and responsibilities further fortify data privacy measures, preventing unauthorized access to sensitive data.
Privacy-enhancing techniques also contribute to overcoming data privacy concerns in AI-driven database management. Techniques such as differential privacy, which injects noise into data to protect individual information while still enabling meaningful analysis, strike a balance between extracting insights and preserving privacy. These mechanisms collectively form a comprehensive approach to safeguarding sensitive data in the age of AI integration.
While the benefits of AI in database management are substantial, the complexity associated with implementing AI models poses a notable challenge. The deployment and maintenance of intricate AI models demand specialized knowledge and resources, potentially acting as barriers to widespread adoption. This complexity is particularly pronounced when dealing with large datasets, intricate algorithms, and the need for continuous optimization.
To navigate this complexity, strategies for simplifying the deployment and maintenance of AI models are imperative. Automated deployment tools and frameworks facilitate a smoother integration process, reducing the burden on database administrators. Containerization technologies, such as Docker, offer a portable and consistent environment for deploying AI models across various systems, streamlining the deployment lifecycle.
Continuous monitoring and optimization mechanisms are equally crucial in managing the complexity of AI models post-deployment. Implementing robust monitoring tools allows for real-time insights into model performance, enabling administrators to identify and address potential issues promptly. The integration of automated optimization processes ensures that AI models evolve alongside changing data dynamics, maintaining their efficacy over time.
In essence, overcoming the complexity associated with AI models in database systems involves a combination of automated deployment tools, containerization, and continuous monitoring. By streamlining the deployment and maintenance processes, organizations can unlock the full potential of AI in database management without succumbing to the challenges posed by model intricacies. As we delve into these strategies, it becomes evident that addressing data privacy concerns and managing model complexity are pivotal aspects of harnessing the transformative power of AI in the realm of database management.
Conclusion
In wrapping up this exploration of the intricate interplay between Artificial Intelligence (AI) and modern database management, a clear narrative emerges — the integration of AI is not a fleeting trend but a transformative force reshaping the very foundations of data management. The embrace of AI in database systems heralds a new era characterized by unparalleled efficiency, fortified security, and groundbreaking innovation, collectively shaping the trajectory of the future in data management.
The symbiotic relationship between AI and modern databases goes beyond mere automation; it signifies a profound shift in the way data is handled, processed, and utilized. The efficiencies gained from AI-driven automation in routine tasks liberate human resources to focus on more strategic and value-driven decision-making. Automation of backups, updates, and maintenance tasks not only ensures reliability but also frees up valuable time for administrators, fostering a more agile and responsive data management ecosystem.
The application of AI in query optimization introduces a level of intelligence that goes beyond conventional approaches. By analyzing and enhancing SQL queries, AI optimizes data retrieval processes, contributing to a more streamlined and efficient database performance. This not only enhances user experience but also positions databases to meet the demands of today's dynamic and data-centric landscape.
Machine learning, a subset of AI, brings predictive analytics into play, allowing database administrators to anticipate future trends and proactively address potential performance bottlenecks. This forward-looking approach enhances the overall efficiency of databases, ensuring that they can dynamically adapt to changing data patterns and user behaviors.
Moreover, the integration of AI in database security introduces adaptive measures that evolve in response to emerging threats. Machine learning algorithms, capable of discerning normal from anomalous activities, provide a robust defense mechanism against ever-evolving cybersecurity challenges. This adaptive security posture ensures that sensitive data remains protected, addressing a critical concern in an era of increasing data breaches and cyber threats.
As we peer into the future, the synergies between AI and quantum computing hold promise for unprecedented data processing power. The concept of autonomous databases, where AI takes the lead in managing, optimizing, and securing databases without continuous human intervention, points towards a future marked by increased operational efficiency and reduced administrative burden.
In conclusion, the integration of AI in modern database management is not a fleeting trend; it is a transformative force steering the course of data management into uncharted territories. The efficiency gains, security enhancements, and innovative possibilities unlocked by AI underscore its pivotal role in shaping the future of data management. As organizations and industries increasingly recognize the potential of this dynamic alliance, the era of AI-driven database management is poised to leave an indelible mark on the evolving landscape of technology and information management.